For most businesses that rely on demand forecasts for supply and capacity planning, improving demand forecast accuracy is critical. There are many methods to measure forecast bias and the accuracy of supply chain forecasts including using statistical methods like the Mean Absolute Percent Error or MAPE that we’ve discussed in our previous blogs. In this blog, I’d like to analyze how including a zero forecast can affect demand forecast accuracy.
Improving demand forecast accuracy: what’s the impact of a zero forecast?
Demand planners often work with sparse datasets. Consider forecasting the sales of a product, at the product customer level, that a customer buys only occasionally. For these types of products, there are bound to be days or months in the forecast where the forecasted number is zero as a result of a no sale.
Here’s the glaring question: What is the impact of including a zero forecast and a corresponding zero shipment when evaluating forecast accuracy?
Let’s consider a sparse dataset that looks like this.
The table below shows a more detailed selection from the dataset that we will analyze.
Let us, first, consider the accuracy of this dataset, with the 0-0 records, included. We will look at the percentage of customer-product pairs forecast within 5% of the volume ultimately shipped.
[Read: Three Steps to a Better Statistical Forecast Setup]
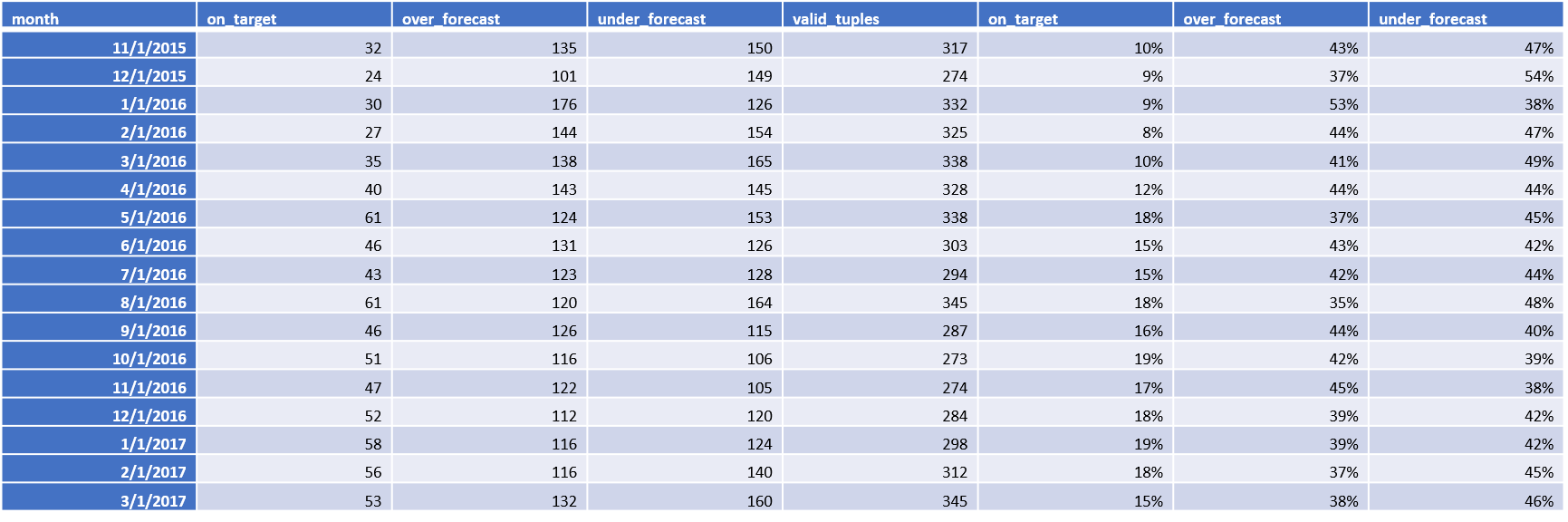
Clearly, excluding the 0-0 records has brought down the average number of records under consideration from 910 to 310. The 600 tuples lost were all classified on_target, which came down from 645 to 45. The number of tuples under_forecast remained at 137; the number of tuples over_forecast at 128. The percentage breakdown of on_target : under_forecast : overforecast changed from 70 : 14 : 15 to 15 : 41 : 44.
Notwithstanding the fact that this is an extreme example, the disparity in the numbers begs the question – should we include 0-0 records?
[Read: How to Report Forecast Accuracy to Management]
Arguments for and against including a zero forecast
The argument against including 0-0 pairs is that the demand planner probably did not actively forecast these customer-product pairs. Including them gives us a misleading measure of forecast accuracy. The pairs might even include customers who no longer buy from the business and obsolete products.
The counter argument is that the 0 forecast may be an active forecast. If the forecaster considered the customer-product combination and forecast, there would be no sale. On the contrary, some non-0 forecasts could be passive. The forecaster simply lets the base forecast numbers go through. We have no way of knowing.
A more pragmatic approach is to consider the purpose of the forecast. If the 0-0 forecast helps us plan production more effectively, we ought to include it. If however, we base our plans on the propensity of the demand planner to hit the mark, we might want to exclude the 0-0 pairs. An example of such a situation would be the fashion industry, where we have a new set of products every season, with no real substitutes that can be re-aligned. Production plans must be made entirely on the estimates offered by a group of buyers.
Do you include or exclude zero forecasts? How does this affect your demand forecast accuracy?
Like this blog? Follow us on LinkedIn or Twitter and we will send you notifications on all future blogs.